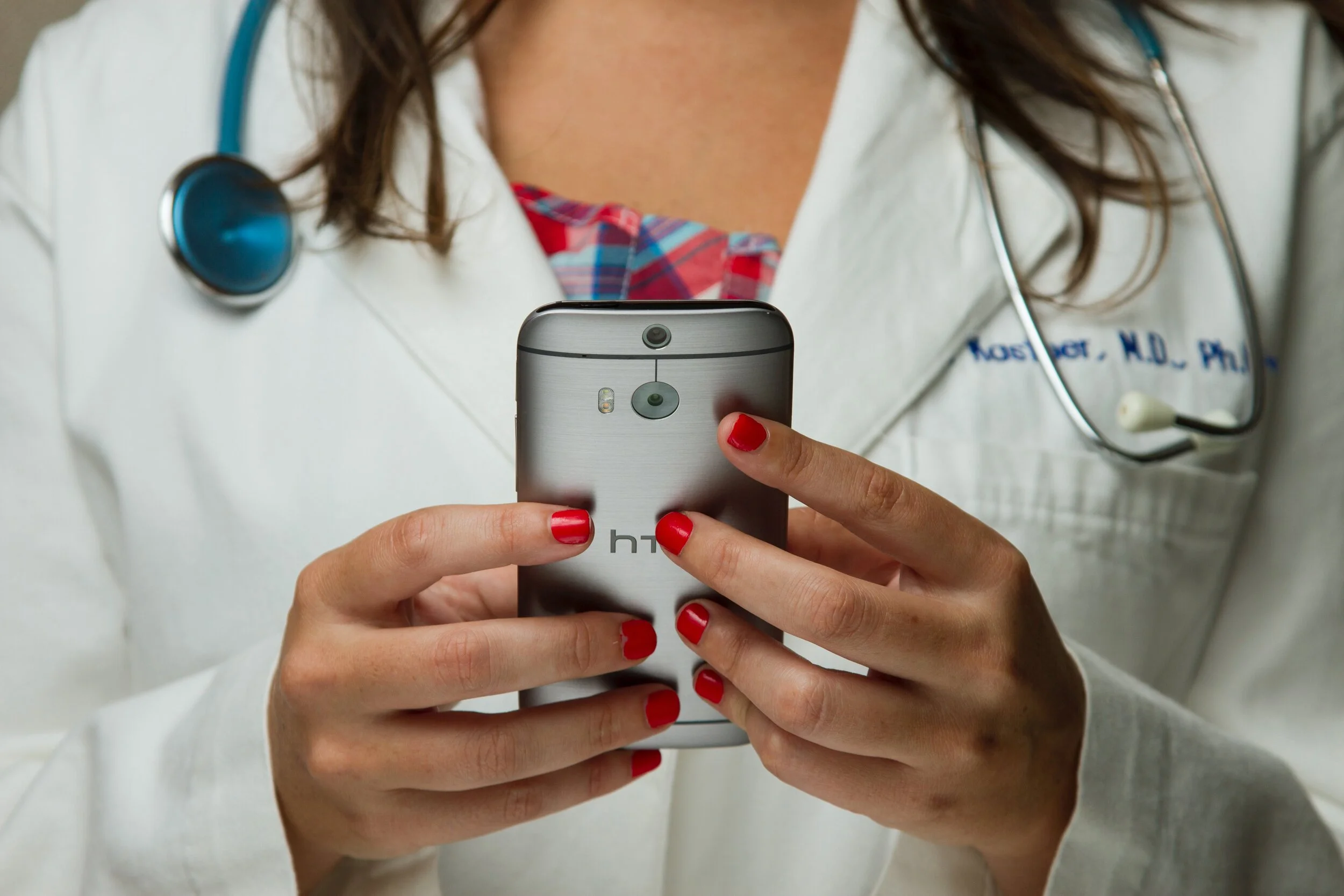
Current State of AI in Healthcare
While we have touched on a lot of information about AI models, ultimately, how can you start applying this knowledge to help you sift through all the news and data about emerging AI tools and applications in healthcare?
The following 3 key questions can be used as a quick method to gain a high level understanding of the potential clinical utility of a model.
Do the training data match the type of data the model uses to make inferences when deployed?
Do the data the model uses when deployed, align with the data that makes the most sense based on the clinical practice workflow in which the model is being used?
Is the model intuitive and does it provide value to the end-user based on its role in the workflow?
Current state of AI in health
With the exponentially increasing amount of literature published every year, it is challenging to stay up-to-date on both state-of-the-art AI techniques and emerging AI use cases (i.e. applications). Keep in mind, possibility does not equal reality. The story of AI in healthcare is riddled with proprietary algorithms, slow adoption, and challenges to implementation. In 2018, there were ~6,000 publications on AI in healthcare, while a recent article outlined only 32 major examples of AI currently in use in healthcare.
The popularity of different AI techniques has evolved and cycled over the years. To provide an in-depth look at every AI-powered technology currently being implemented or explored in healthcare would be beyond the capabilities and the scope of this series. Our intent is to provide you with a high level overview of the current state of AI in healthcare and to do so, we have chosen several key examples to illustrate existing AI capabilities and trends in healthcare.
Applications and use cases — Biopharmaceuticals Industry
Drug discovery
Companies leading this area are looking at drug discovery from multiple angles. For example, one start-up is amassing data from research papers, patents, clinical trials, and patient records to delineate potential relationships between genes, proteins, diseases, candidate drugs, and other biological elements.¹ The goal is to use multiple machine learning techniques, including NLP, in order to find new patterns by combining different types of information spread across numerous sources and domains.¹ These insights can then be used to inform methods to better target disease processes.
Drug re-purposing
The traditional process for drug discovery involves high throughput screening (HTS) of large compound libraries. By today's standards, HTS is an inefficient, costly, and arduous process. Artificial intelligence has emerged as an approach that wins on accuracy and efficiency; because AI models can find patterns in data, they are able to sift through the compounds in a manner that makes scientific and clinical sense. Putting this technology to work in a compound library of already approved drugs can help researchers identify molecules that are fit for repurposing, while also doing so at a much faster rate than humans.
A recent study evaluated a deep learning approach for in silico identification of molecular targets from existing drug compounds. deepDTnet, as it is called, draws from chemical, genomic, phenotypic, and cellular information to determine biological and pharmacological relationships.² From their algorithm, they are able to profile the vast drug-target interactions of each drug molecule which often contribute to 'off-target side effects', but in this case, may have potential for treating other disease states.
Yet another example would be Pharnext, a startup born ahead of its time, headed by Daniel Cohen, who helped produce the first-ever human genetic linkage map. They recently demonstrated positive results in a Phase III trial with a drug combination made up of already available drugs, baclofen and naltrexone.³ This compound, PXT3003, aims to treat Charcot-Marie-Tooth disease, a rare neurodegenerative condition, and has been granted fast-track status by the FDA.³
Clinical trials
Clinical trials can be a painstakingly slow undertaking, which is exacerbated by multiple bottlenecks that exist early on in the process. One of the largest bottlenecks is the patient recruitment process, which is both time-consuming and costly. Oftentimes, fewer than the goal number of patients are found, which delays the start of the clinical trial tremendously. Fortunately, technology is able to assist. With the amount of data that resides in the healthcare industry, digital tools such as artificial intelligence and machine learning, can help optimize the process by picking out the most relevant information to act upon. If we look at the recruitment process, this would mean having an AI model predetermine the most suitable candidates within a particular disease state who are most likely to respond to a candidate drug.⁴ From the patient's perspective, this could also simplify their search for an appropriate clinical trial. The below image depicts additional areas of the clinical trial process in which AI can be leveraged.
From the IQVIA Report "The Changing Landscape of Research and Development," April 2019 (Advanced Analytics models simply refer to Machine Learning models)
Applications and use cases — Direct Patient Care
Predictive models
Predictive modeling involves scanning historical data to predict future outcomes, and it can be applied across a range of healthcare operations, from enabling earlier diagnosis to optimizing hospital triage for patients. It allows clinicians to shift from traditional reactive care to a proactive approach. In an industry in which data are abundant, AI is transforming the way we build and use predictive models, moving us towards greater individualized care. It is anticipated that this technology will improve the way diagnostics, prescriptions, and treatments are handled. Below are a few examples:
Palliative Care: Penn Medicine developed a system, called Palliative Connect, which is a mortality prediction model. Leveraging clinical data from electronic health records, it aims to identify hospitalized patients who are at risk for poor health outcomes and would, therefore, benefit from early palliative consultation.⁵ Additionally, NLP can be used to examine the content and sentiment of ICU clinical notes to predict mortality risk in patients; use of NLP-based methods performed better for mortality prediction than using structured clinical data alone.⁶
Chronic Kidney Disease: Cricket Health is a startup focused on kidney care. Armed with a multi-year dataset that includes information such as demographics and patient co-morbidities, the team developed a machine learning model to identify patients at-risk for or living with chronic kidney disease and to predict an estimated glomerular filtration rate for those patients.⁷
Oncology: A significant challenge in the field of pathology is the heterogeneity that exists among pathologists' interpretation of biopsies. One can imagine how this may lead to negative downstream effects relating to the patient's treatment. Current use cases of predictive analytics in this field use tumor characteristics to predict prognosis and response to therapy.⁸
Risk of Opioid unintentional overdose death: NarxCheck Score uses machine learning to predict risk, at the individual-level, of death from an unintentional opioid overdose. The score was designed to help guide clinician decision-making in real time.⁹
Diagnostics
IDx-DR is the first AI model approved by the FDA to diagnose autonomously (i.e. without human intervention).¹⁰ IDx-DR uses its software to analyze retinal images of the eye for the presence of more-than-mild diabetic retinopathy (mmDR).¹¹ Currently, the AI-powered software is only approved for use with the Topcon NW400 fundus camera.¹¹ Similar to medications requiring approval for additional indications, IDx-DR must submit to the FDA for approval of any additional camera models they would like to use with their software. As mentioned previously, because cameras can produce images of different size, resolution, and noise, the model has to be trained on and/or validated with images specific to each camera for which it will be used.
Radiology
There are many examples of companies, such as Arterys, Zebra Medical Vision, or qure.ai who provide AI-enabled diagnostic suggestions, abnormality detection, or predictions based on radiographic imaging. As a task well suited for deep learning, image recognition in healthcare is a growing field. A key difference in these applications compared to IDx-DR is that they do not operate autonomously.
Voice analytics
Machine learning can be used to detect the likelihood someone has a disease or condition based on the sound of their voice.¹² It looks at the audio data and can discern patterns that would be undetectable to the human ear. Several startups, including Vocalis Health (previously, Beyond Verbal) and Sonde Health, are exploring voice analysis to inform disease diagnosis; specifically, these companies are exploring association of voice with depression, respiratory health, and emotional health.¹³
Drug recommendations
AI may be able to help optimize drug therapy selection. Treatment with antidepressants frequently yields low response rates and there is often a need to trial several medications before seeing efficacy. As a result, we are constantly trying to identify better ways to understand who might respond to which medication, and AI has opened up new avenues to explore. Researchers have found that AI can help predict antidepressant response based on an individual’s electroencephalography profile, which is a measurement of the brain’s electrical activity.¹⁴
Pharmacogenomics (PGx) is an emerging field that explores the affect of an individual's genes on their response to drugs. As it stands today, we have accumulated vast amounts of data on drug-gene interactions, but only for a small number of those interactions do we have actionable recommendations to follow (e.g. increase dose by 25% for ultra-rapid metabolizers; or contraindicated in individuals with loss-of-function metabolizing genes). There are significant challenges with antidepressant therapy owing to the heterogeneity of psychiatric disorders among patients. Athreya et al. explored the integration of machine learning with validated PGx single nucleotide polymorphism (SNP) biomarkers, which accurately predicted the 8-week treatment response to two SSRI antidepressants, citalopram and escitalopram.¹⁵ Their research highlights that leveraging machine learning methods in PGx analysis can help to improve treatment choice, and ultimately, efficacy and safety outcomes for patients. When we look at direct patient care, PGx has implications in medication optimization, but from the perspective of the biopharmaceutical industry, it remains an untapped area within drug discovery and development.
Clinical decision support
Unsupervised learning models built around anomaly detection are being used to help develop clinical decision support (CDS) tools, including drug alert notifications in the EHR. Due to the limitations of current rules-based CDS tools, such as alert fatigue, there is a growing need for more "intelligent", dynamic, and adaptable CDS technology.
MedAware, for example, has built a CDS tool which identifies potentially inappropriate medication orders based on what is typically ordered for similar patients; it does so by looking at the patient’s clinical status, baseline characteristics, and medical history.¹⁶ If the medication order — whether the drug, route, or dose — deviate significantly from what is commonly ordered for similar patients, an alert will pop up to advise the user to double-check the order. It also provides the percentages of medication(s) that are most commonly seen ordered in a given situation for similar patients, in case the user accidentally entered the wrong medication or perhaps missed a key patient lab, such as a hepatic or renal function indicator. This model is predicated on the idea that the most common therapeutic interventions are appropriate and something that deviates from that could be an error. However, as we are aware, this is not always guaranteed to be the case. There are always exceptions to the rule.
Wearables
Wearables are becoming more and more prevalent in society today and have rapidly taken off amongst consumers. In healthcare, these devices employ a number of algorithms to assist the user in detecting, tracking, and monitoring biometric data. Wearables can also be used to augment physiological functions. OrCam MyEye, for example, intended for visually impaired individuals, is one that, when attached to eyeglasses, will help with reading text and facial recognition.¹⁷ Livio AI, developed by Starkey Hearing Technologies, is a hearing aid product that leverages natural language processing to translate speech for people with hearing impairments.¹⁷ Other wearables currently in the market monitor biometric data such as heart rate and ECG; their utility is in providing real-time, continuous data and, depending on the purpose and regulation-status of the device, helping inform diagnostics, treatment, and patient outcomes.¹⁸ Many may be aware of innovative continuous glucose monitoring systems such as the Freestyle Libre, the data of which, if combined with laboratory values, blood work, patient demographics, and other pertinent health data, can be used for algorithm development, with the goal of improving treatment paradigms.
Adherence
Companies like AiCure use facial recognition and deep learning to monitor patient adherence to investigational drugs. When a patient in a study is about to take a dose of their medication, for example, the patient would open the AiCure app on their phone and hold the camera towards their face. The app uses image recognition to confirm patient identity and analyzes patient movements to confirm that the medication was ingested. The company also uses machine learning techniques to monitor facial expressivity and capture level of patient engagement.
Chat Bots
Chat Bots allow companies to create new and scalable interfaces for interacting with patients and providing important, current, and relevant medical information or education. Although they have been used in industries outside of healthcare for a while, healthcare is beginning to see more adoption as the technology improves. Common use cases for health-related chat bots include diagnostic symptom checkers, triage applications, and applications designed to provide clinical drug information.
How to stay current and stay smart
As mentioned in our Introduction post, we need to differentiate between hype and reality. Revisiting the concept of AI winters, it's important to understand the role that public opinion plays on AI marketing and terminology. During an AI winter, when there is a decrease in general interest in AI, it is often accompanied by changes in marketing and branding to offset public perception. For example, the term “AI” might be replaced by other terms, such as “cognitive computing” or “cognitive machines.” Some companies have already made the decision to avoid using the term “artificial intelligence” to describe their operations in anticipation of the possibility of a shift in public opinion and the onset of another AI winter. Other companies, meanwhile, are capitalizing on the current hype cycle and branding everything as “AI-powered." However, as we have seen, not all AI models are the same. Deep learning models are very different from logistic regression, but both could be labelled by a company as state-of-the-art artificial intelligence tools. Ultimately, it is important to keep the following in mind:
Just because there is a lot of hype around AI, currently, does not mean that it will be able to live up to potentially inflated expectations (as evidenced by its rocky history). Be skeptical of any too-good-to-be-true claims about AI’s ability.
Never take a company’s (or researcher’s) claim of using AI/ML in healthcare at face value. "Artificial intelligence" by itself is not specific enough and should be accompanied with a description about the type of AI model or methodology used. Be cautious about companies or articles that do not provide those details.
Referenes:
Fleming N. How artificial intelligence is changing drug discovery. Nature. 2018;557(7707):S55-S57.
Keshavarzi Arshadi A, Salem M, Collins J, Yuan JS, Chakrabarti D. DeepMalaria: Artificial Intelligence Driven Discovery of Potent Antiplasmodials. Front Pharmacol. 2019;10:1526.
Zeng X, Zhu S, Lu W, et al. Target identification among known drugs by deep learning from heterogeneous networks. Chem Sci. 2020;11(7):1775-1797.
Woo M. An AI boost for clinical trials. Nature. 2019;573(7775):S100-S102.
Courtright KR, Chivers C, Becker M, et al. Electronic Health Record Mortality Prediction Model for Targeted Palliative Care Among Hospitalized Medical Patients: a Pilot Quasi-experimental Study. J Gen Intern Med. 2019;34(9):1841-1847.
Jin M, Bahadori MT, Colak A, et al. Improving Hospital Mortality Prediction with Medical Named Entities and Multimodal Learning. arXiv [csCL]. November 2018. http://arxiv.org/abs/1811.12276.
Cricket Health News. Cricket Health Announces New Machine Learning Model for Detecting Chronic Kidney Disease – Cricket Health. Cricket Health. https://www.crickethealth.com/cricket-health-announces-new-machine-learning-model-for-detecting-chronic-kidney-disease/. Published November 6, 2019.
Parikh RB, Gdowski A, Patt DA, Hertler A, Mermel C, Bekelman JE. Using Big Data and Predictive Analytics to Determine Patient Risk in Oncology. Am Soc Clin Oncol Educ Book. 2019;39:e53-e58.
Huizenga JE, Breneman BC, Patel VR, A. R, Speights DB. NARxCHECK Score as a Predictor of Unintentional Overdose Death. https://apprisshealth.com/wp-content/uploads/sites/2/2017/02/NARxCHECK-Score-as-a-Predictor.pdf.
DeNovo Summary 180001. https://www.accessdata.fda.gov/cdrh_docs/reviews/DEN180001.pdf.
Marmar CR, Brown AD, Qian M, et al. Speech-based markers for posttraumatic stress disorder in US veterans. Depress Anxiety. 2019;36(7):607-616.
Brown B. Voice Analysis to Diagnose and Monitor Health. Health Tech Insider. https://healthtechinsider.com/2017/12/13/voice-analysis-diagnose-monitor-health/. Published December 13, 2017.
Jaworska N, de la Salle S, Ibrahim M-H, Blier P, Knott V. Leveraging Machine Learning Approaches for Predicting Antidepressant Treatment Response Using Electroencephalography (EEG) and Clinical Data. Front Psychiatry. 2018;9:768.
Athreya AP, Iyer R, Wang L, Weinshilboum RM, Bobo WV. Integration of machine learning and pharmacogenomic biomarkers for predicting response to antidepressant treatment: can computational intelligence be used to augment clinical assessments? Pharmacogenomics. 2019;20(14):983-988.
Rozenblum R, Rodriguez-Monguio R, Volk LA, et al. Using a Machine Learning System to Identify and Prevent Medication Prescribing Errors: A Clinical and Cost Analysis Evaluation. Jt Comm J Qual Patient Saf. 2020;46(1):3-10.
de Jesus A. Using Wearable Data for Artificial Intelligence Applications – Current Use Cases | Emerj. Emerj. https://emerj.com/ai-sector-overviews/using-wearable-data-for-artificial-intelligence-applications-current-use-cases/.
Tran V-T, Riveros C, Ravaud P. Patients’ views of wearable devices and AI in healthcare: findings from the ComPaRe e-cohort. NPJ Digit Med. 2019;2:53.